-
Notifications
You must be signed in to change notification settings - Fork 68
Commit
This commit does not belong to any branch on this repository, and may belong to a fork outside of the repository.
Signed-off-by: YunLiu <[email protected]>
- Loading branch information
Showing
1 changed file
with
103 additions
and
0 deletions.
There are no files selected for viewing
This file contains bidirectional Unicode text that may be interpreted or compiled differently than what appears below. To review, open the file in an editor that reveals hidden Unicode characters.
Learn more about bidirectional Unicode characters
Original file line number | Diff line number | Diff line change |
---|---|---|
@@ -0,0 +1,103 @@ | ||
# Model Overview | ||
A pre-trained model for volumetric (3D) segmentation of the spleen from CT images. | ||
|
||
This model is trained using the runner-up [1] awarded pipeline of the "Medical Segmentation Decathlon Challenge 2018" using the UNet architecture [2] with 32 training images and 9 validation images. | ||
|
||
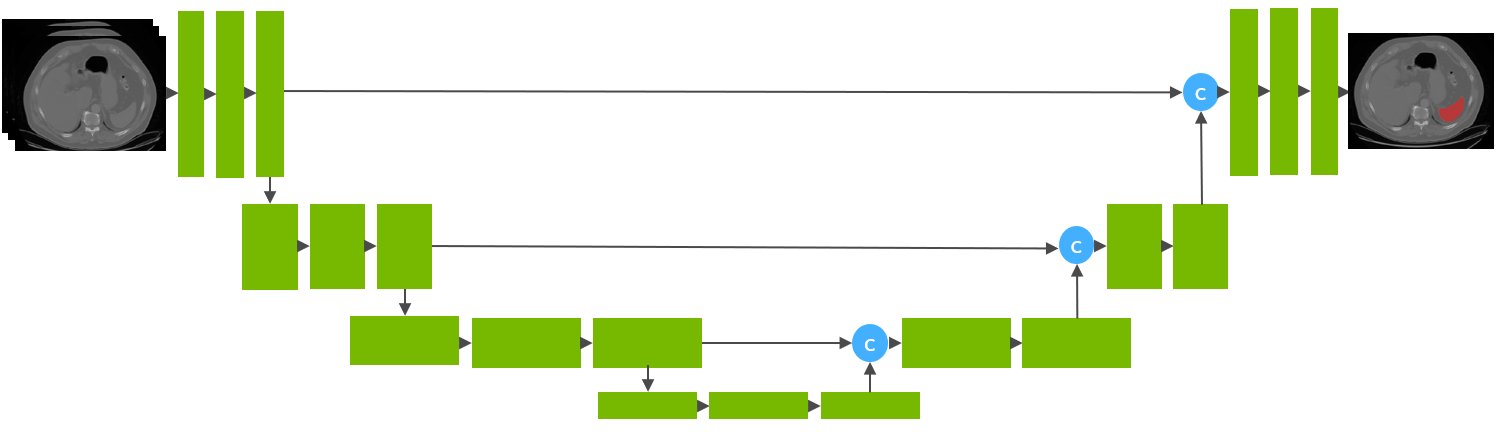 | ||
|
||
## Data | ||
The training dataset is the Spleen Task from the Medical Segmentation Decathalon. Users can find more details on the datasets at http://medicaldecathlon.com/. | ||
|
||
- Target: Spleen | ||
- Modality: CT | ||
- Size: 61 3D volumes (41 Training + 20 Testing) | ||
- Source: Memorial Sloan Kettering Cancer Center | ||
- Challenge: Large-ranging foreground size | ||
|
||
## Training configuration | ||
The segmentation of spleen region is formulated as the voxel-wise binary classification. Each voxel is predicted as either foreground (spleen) or background. And the model is optimized with gradient descent method minimizing Dice + cross entropy loss between the predicted mask and ground truth segmentation. | ||
|
||
The training was performed with the following: | ||
|
||
- GPU: at least 12GB of GPU memory | ||
- Actual Model Input: 96 x 96 x 96 | ||
- AMP: True | ||
- Optimizer: Novograd | ||
- Learning Rate: 0.002 | ||
- Loss: DiceCELoss | ||
- Dataset Manager: CacheDataset | ||
|
||
### Memory Consumption Warning | ||
|
||
If you face memory issues with CacheDataset, you can either switch to a regular Dataset class or lower the caching rate `cache_rate` in the configurations within range [0, 1] to minimize the System RAM requirements. | ||
|
||
### Input | ||
One channel | ||
- CT image | ||
|
||
### Output | ||
Two channels | ||
- Label 1: spleen | ||
- Label 0: everything else | ||
|
||
### Typical Usage: Real-Time Inference Execution | ||
|
||
The following example demonstrates how to execute real-time inference using both Pythonic and config-based bundles with MONAI: | ||
|
||
``` | ||
from monai.bundle import create_workflow | ||
from monai.transforms import LoadImaged | ||
from monai.data import CommonKeys # Ensure proper imports | ||
# Pythonic bundle workflow creation | ||
workflow = create_workflow("inference.InferenceWorkflow") | ||
# Config-based workflow creation | ||
workflow = create_workflow(config_file="./inference.json") | ||
# Initialize the workflow | ||
workflow.initialize() | ||
# Load input data | ||
input_loader = LoadImaged(keys="image") | ||
workflow.dataflow.update(input_loader({"image": "/workspace/Data/Task09_Spleen/imagesTr/spleen_46.nii.gz"})) | ||
# Run the inference | ||
workflow.run() | ||
# Update dataflow with new input | ||
workflow.dataflow.clear() | ||
workflow.dataflow.update(input_loader({"image": "/workspace/Data/Task09_Spleen/imagesTr/spleen_38.nii.gz"})) | ||
# Run the inference again | ||
workflow.run() | ||
# Retrieve the output | ||
output = workflow.dataflow[CommonKeys.PRED] | ||
print(f"Inference Output: {output}") | ||
# Finalize the workflow | ||
workflow.finalize() | ||
``` | ||
|
||
|
||
|
||
# References | ||
[1] Xia, Yingda, et al. "3D Semi-Supervised Learning with Uncertainty-Aware Multi-View Co-Training." arXiv preprint arXiv:1811.12506 (2018). https://arxiv.org/abs/1811.12506. | ||
|
||
[2] Kerfoot E., Clough J., Oksuz I., Lee J., King A.P., Schnabel J.A. (2019) Left-Ventricle Quantification Using Residual U-Net. In: Pop M. et al. (eds) Statistical Atlases and Computational Models of the Heart. Atrial Segmentation and LV Quantification Challenges. STACOM 2018. Lecture Notes in Computer Science, vol 11395. Springer, Cham. https://doi.org/10.1007/978-3-030-12029-0_40 | ||
|
||
# License | ||
Copyright (c) MONAI Consortium | ||
|
||
Licensed under the Apache License, Version 2.0 (the "License"); | ||
you may not use this file except in compliance with the License. | ||
You may obtain a copy of the License at | ||
|
||
http://www.apache.org/licenses/LICENSE-2.0 | ||
|
||
Unless required by applicable law or agreed to in writing, software | ||
distributed under the License is distributed on an "AS IS" BASIS, | ||
WITHOUT WARRANTIES OR CONDITIONS OF ANY KIND, either express or implied. | ||
See the License for the specific language governing permissions and | ||
limitations under the License. |